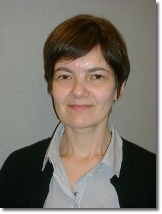
Dina Demner Fushman
NIH/NLM/LHC
USA
Multimodal Biomedical Information Retrieval
[Abstract]The search for relevant and actionable information is key to achieving clinical and research goals in biomedicine. Biomedical information exists in different forms: as text and illustrations in journal articles and other documents, in images stored in databases, and as patients' cases in electronic health records. This talk will present ways to move beyond conventional text-based searching of these resources by combining text and visual features in search queries and document representation. A combination of techniques and tools from the fields of Natural Language Processing, Information Retrieval, and Content-Based Image Retrieval allows to develop building blocks for advanced information services. Such services will enable searching by textual as well as visual queries, and retrieving documents enriched by relevant images, charts, and other illustrations from the journal literature, patient records and image databases.
[Speaker's Bio]
Dina Demner-Fushman is a Staff Scientist for the Communications Engineering Branch at the National Library of Medicine. She conducts research in clinical decision support, clinical question answering, use of natural language processing in information retrieval, human computer interaction aspects of information retrieval, and information retrieval in biomedical domain. Her interest in biomedical language processing stems from years of clinical practice (M.D. obtained from Kazan State Medical Institute in 1980) and clinical research (Doctorate (Ph.D.) in Medical Science earned from Moscow Medical and Stomatological Institute in 1989.) She earned her MS and PhD in Computer Science from the University of Maryland, College Park in 2003 and 2006, respectively. She earned her B.S degree in Computer Science from Hunter College, CUNY in 2000.
Can machines understand chemistry in scientific publications?
[Abstract]Many papers in lifesciences contain significant numbers of chemical concepts. These range from mentions of chemical entities to descriptions of procedures largely based on chemistry (synthesis, analysis, activity). We have developed a package of Open Source tools to cover many of the requirements of life scientists.
Chemical entity recognition: The OSCAR program (now V4) recognises chemical entities in free text. It uses a variety of processes: firstly tokenization (including chemistry-specific rules); then recognizers (using some or all of POS tagging, n-grams, machine learning, lookup, and heuristics). Chemical language can be found in a wide variety of styles (articles, abstracts) and OSCAR has been developed so that it can be retrained for different subdomains.
Chemical name interpretation: many compounds are described using systematic (IUPAC) chemical names and OPSIN analyses these through an automaton-based system. Its success is very high (97% recall and 99.7% accuracy). OPSIN is designed so that it can be extended to semi-synthetic names and other human languages.
Chemical phrase interpretation: Using OpenNLP and the ANTLR grammar tool ChemicalTagger interprets the stock phrases used in chemical synthesis, classifying phrases into ca. 20 categories ("add", "stir", "heat", etc.) In many cases the phrases are completely parsed and the complete recipe can be extracted in semantic form.
The combination of these tools has been applied to parsing chemical patents and in many cases the complete, balanced, chemical reaction (including structure diagrams and stoichiometry) can be extracted solely from the text.
In conjunction with the analysis of structure diagrams in text (using the NIH's OSRA program) we are now technically capable of extracting chemistry from a wide range of life-science publications.
The primary problem facing natural language processing of chemistry is publisher-imposed restrictions on the re-use of published science. It is very difficult to build corpora and training sets that can be redistributed Openly. Similarly mass extraction of chemical information, even by subscribers, is explicitly prohibited. I shall present aspects of this problem to the conference.
[Speaker's Bio]
Peter Murray-Rust, originally a crystallographer with a DPhil from Oxford, is Reader in Molecular Informatics at the University of Cambridge and Senior Research Fellow of Churchill College. His interests have involved the automated analysis of data in scientific publications, creation of virtual communities e.g. The Virtual School of Natural Sciences in the Globewide Network Academy and the Semantic Web. With Henry Rzepa he has extended this to chemistry through the development of Markup languages, especially Chemical Markup Language. He is leading several research projects such as OSCAR, OPSIN and ChemicalTagger, to develop Natural Language Processing to extract chemistry and other physical science from traditional publications. He serves on the advisory board of UKPubmedCentral, which offers free access to a vast and growing collection of biomedical and health research information. He campaigns for Open Data, particularly in science, and is on the advisory board of the Open Knowledge Foundation. Together with a few other chemists he was a founder member of the Blue Obelisk movement in 2005.

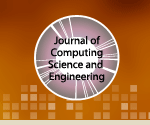
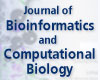